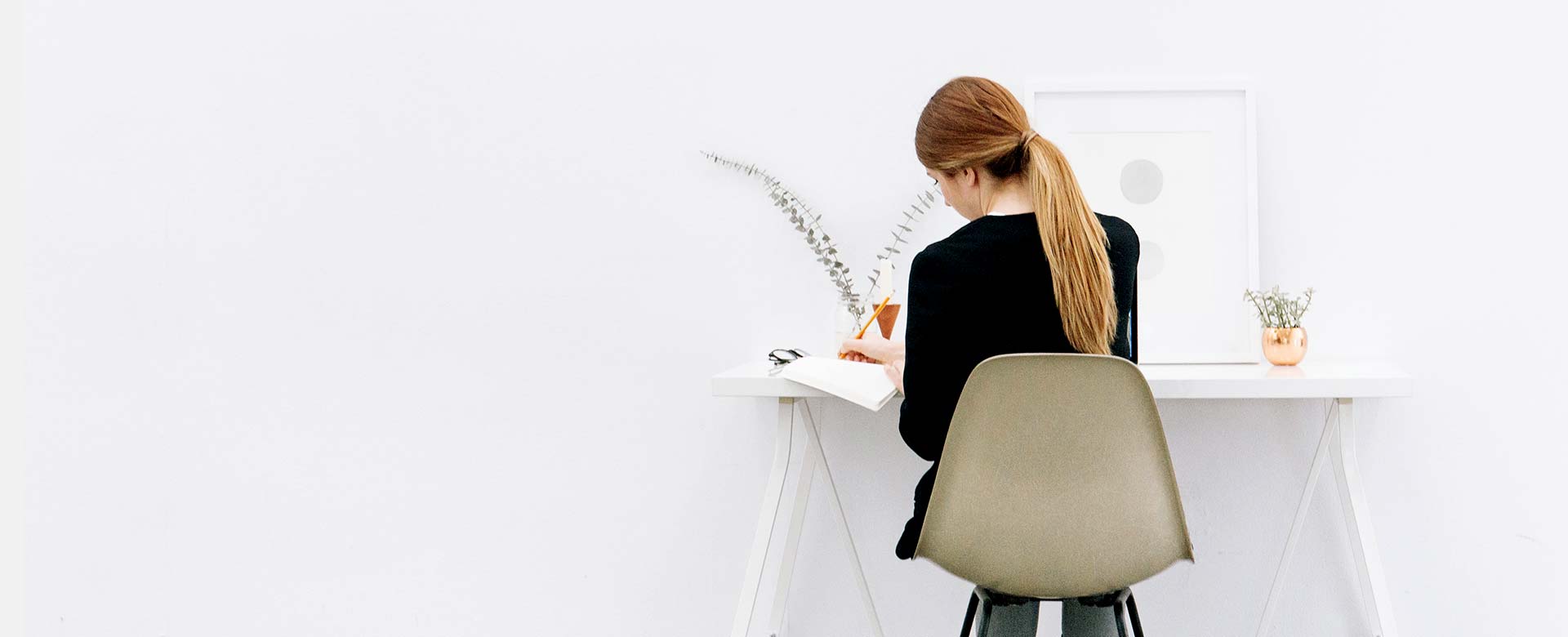
Why bother with Data?
That is the question that almost every tech company has found the answer to and are scrambling to find people who can make sense of the data. The answer is pretty simple: Predictions. But the solution is not as easy. We have to collect the data, clean it, analyze it, find patterns, build models, test the models, refine the models and then make predictions with acceptable chances of messing up. In essence, that is all that there is to it. I tried to explain concept of prediction from the data, to the nurse giving me flu-shot, and she just accused me wanting to play God. But, are we really? Are we trying to play God, using all the data? Maybe. A little bit. If God is happy with being 85% sure about something happening.
Pattern recognition and predictions based on previous occurrences are not new technology. It has been written into our genetic code as we evolved from primitive forms. Our human pattern recognition is so good that our brain does it effortlessly. In fact, it is incredibly good that many a times finds patterns even if it's not there. We could find the face of God in a piece of food and assume that God has arrived to redeem us and quit our jobs to go to a place of worship or our statistical model could fit the previous day's stock market data perfectly and we scrape all our money and invest based that model; either would end up vastly detrimental for us. In humans we call it Pareidolia and in statistical models we call it over-fitting; they are both cases of Apophenia, finding meaning and patterns when it doesn't exist.
Humans are creatures of pattern. We follow a pattern in our everyday routine. With enough data, and a good statistical model we could predict a person's specific activity with a certain level of confidence. If the person deviates a little from the previously known activity, those could be outliers. But if the person deviates all the time from the previous pattern, then that becomes the new pattern and we have to update our model. Humans use patterns and prediction to improve efficiency, by nature.
As an example through story time: My mom used to usually call me out three to four times saying the dinner was ready and I would go after some time (Yes, I was a spoilt child!). One day, I decided to be nice and I just went down right after the first time she said the food was ready. To my surprise, she wasn't even done cooking! She had used my previous behavior and predicted that I would be late and adjusted her timeline based on that. All prediction models are subject to errors. The impact of the error is trivial in the case of dinner time. But other applications like emergency response team's arrival, medical diagnosis, etc. are highly critical and the prediction error should be minimized as much statistically possible. That is why we study the data and learn to make better predictions and identify patterns better, to make the life better, for the planet and everyone on it.
0 Comments